-
What We Do
-
Feasibility AssessmentMinimize risk, maximize results, and set your organization up for success.
-
AI LabLeverage shared experience and collaboration to drive adoption and results.
-
Catalog of OpportunitiesEmbark on your AI adoption journey with confidence.
-
Generative AI WorkshopUncover innovative ways to engage with your data to shape your future.
-
-
Featured
Navigating Bias in AI with Open-Source Toolkits
-
Some Industries We Support
-
Energy and ResourcesDrive innovation and promote sustainability while gaining a competitive edge.
-
Financial ServicesBoost efficiency, reduce costs, and streamline processes.
-
Forestry and AgriculturePave the way for a productive, efficient, and greener future.
-
HealthRevolutionize care delivery, improve outcomes, and save lives.
-
ManufacturingStreamline production, eliminate costly downtime, and enhance quality control.
-
-
Featured
AltaML Secures Spot on AIFinTech100 for Consecutive Year
-
What We Do
-
Contact
-
Featured
Jurisage and CiteRight Finalize Merger, Become Jurisage Group Inc.
-
Services
-
What We Do
-
Feasibility AssessmentMinimize risk, maximize results, and set your organization up for success.
-
AI LabLeverage shared experience and collaboration to drive adoption and results.
-
Catalog of OpportunitiesEmbark on your AI adoption journey with confidence.
-
Generative AI WorkshopUncover innovative ways to engage with your data to shape your future.
-
-
-
Industries
-
Some Industries We Support
-
Energy and ResourcesDrive innovation and promote sustainability while gaining a competitive edge.
-
Financial ServicesBoost efficiency, reduce costs, and streamline processes.
-
Forestry and AgriculturePave the way for a productive, efficient, and greener future.
-
HealthRevolutionize care delivery, improve outcomes, and save lives.
-
ManufacturingStreamline production, eliminate costly downtime, and enhance quality control.
-
-
-
Venture Studio
-
What We Do
-
Contact
-
- Insights
-
About

Insights
Why Enterprise AI Needs a Data-Product Approach

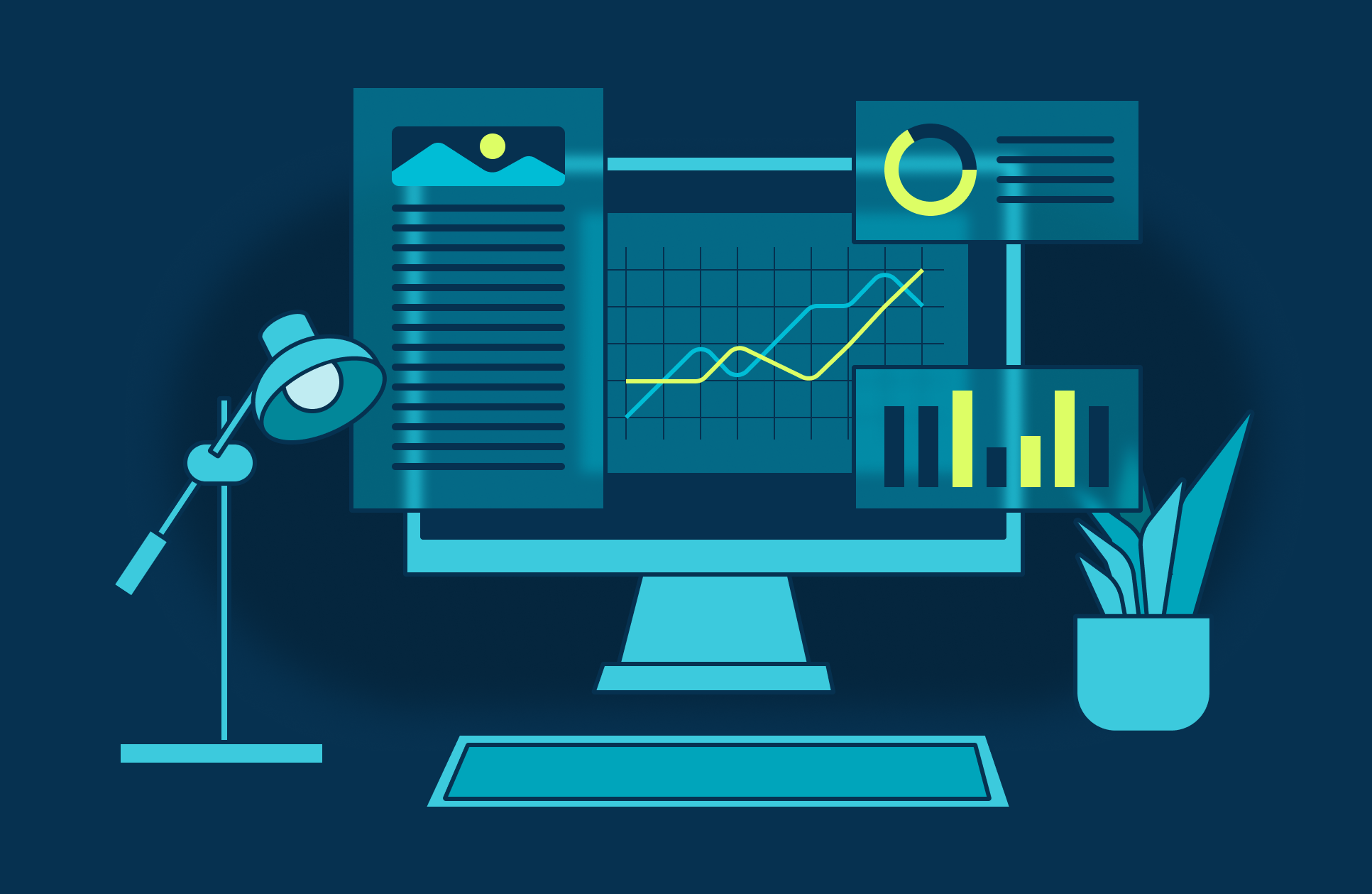
From Data Warehouses to Data Lakes
In the 1990s and early 2000s data warehouses gained a lot of traction. They were used to draw insights from aggregated data organized in multidimensional data structures. It quickly became clear that it was advantageous to think about approaching data warehouse building in a more modular approach with the use of data marts. The data marts were usually focused on a specific business department or data domain.
In more recent times, the surge of big data technology saw data lakes ascend in popularity, largely due to their capacity to incorporate unstructured data from files alongside structured data from databases. Their appeal was also attributed to simplified data loading, foregoing intricate transformations upfront. Intriguingly, my interactions with enterprise clients revealed an interesting observation—while the segmentation methodology applied to data warehouses into data marts seemed to fit seamlessly, the transition wasn’t as immediate for data lakes. There is often an ambition to load every piece of data possible into the data lake before starting to drive insights, which tends to be a huge undertaking, potentially requiring millions of dollars and multi-year efforts, in many cases. It is also often counterproductive—until you start working on a real use case, you may not understand the business processes well enough to know what data is actually valuable.
The Age of AI and Data Products
To work around that challenge, modern approaches saw an inclination toward a use-case-driven strategy in the age of artificial intelligence (AI). Rather than embarking on the complex and time-consuming journey of establishing a data lake first, organizations opted for a pragmatic use-case-by-use-case method. This approach allowed for focused data engineering and preparation efforts aligned with specific AI business use cases. While this is a commendable entry point to AI adoption, it warrants caution as an organization scales the number of AI and machine learning (ML) projects in production. The risk emerges in the form of potential duplicated efforts and inefficiencies, considering that numerous use cases may require the same datasets.
We, along with many others in the industry, believe that there exists an alternative approach—one that bridges the gap between these paradigms. This perspective advocates for viewing data through a product-centric lens, with an emphasis on constructing data products.
What Is a Data Product?
Consider a scenario where a supply chain department has multiple business use cases that promise enhanced efficiency, improved services, and cost savings. It’s plausible that the datasets crucial for these use cases are confined within a finite set of sources, representing a fraction of the entire company’s data lake. The following sequence outlines the high level steps:
- Identify a subset of high-value business use cases for implementation within a specific timeframe, for example, one year.
- Determine the requisite data sources to effectively address these business use cases.
- Identify data stewards, owners, and access protocols governing these data sources.
- Develop pipelines to populate the designated data domain.
- Construct data products on top of the established data domain.
In other words, the definition of data product we use in this context is the combination of data, the associated metadata, code that transforms that data, ML model(s) and the data visualization user interface that a user interacts with to meet their business requirements. Under this definition, data is now treated as a first class citizen and concepts like a data product owner and a data product roadmap are extremely important pieces of the puzzle.
Advantages of a Data-Product Approach
Embracing a data product approach has unveiled several noteworthy advantages:
- While the initial investment and time-to-value for the first business use case may be substantial, subsequent use cases have seen both investment and time-to-value be cut in half.
- There’s a heightened concentration on business value. By shifting focus from “constructing one more ML model” to crafting a data product in which the ML model is only one component alongside dashboards, data visualizations, supplementary rules-based capabilities, and enhanced workflows, we’ve observed an organic shift towards prioritizing end-user experience and a more human-centric design.
- There is greater opportunity for reuse and extensibility. A data product may end up being expanded or combined with other data products to achieve additional business outcomes.
- Concentrating efforts within a single domain over a period facilitates elevated data literacy, engagement, and business backing—a potential catalyst for data-driven innovation across the entire organization. Having internal champions speaking unsolicited about the value they’ve achieved to their peers adds a compelling advantage.
Conclusion
As previously mentioned, this is an approach for which we see increased support in industry. In addition, it is also very much technology and platform agnostic, meaning it can be adopted regardless of the specific hyperscaler and technology stack that an organization may have chosen. As we continue on our path to help clients make their enterprise AI adoption journey less hard, this is an approach that we plan to continue to adopt, refine, and measure the outcomes.