-
What We Do
-
Feasibility AssessmentMinimize risk, maximize results, and set your organization up for success.
-
AI LabLeverage shared experience and collaboration to drive adoption and results.
-
Catalog of OpportunitiesEmbark on your AI adoption journey with confidence.
-
Generative AI WorkshopUncover innovative ways to engage with your data to shape your future.
-
-
Featured
Navigating Bias in AI with Open-Source Toolkits
-
Some Industries We Support
-
Energy and ResourcesDrive innovation and promote sustainability while gaining a competitive edge.
-
Financial ServicesBoost efficiency, reduce costs, and streamline processes.
-
Forestry and AgriculturePave the way for a productive, efficient, and greener future.
-
HealthRevolutionize care delivery, improve outcomes, and save lives.
-
ManufacturingStreamline production, eliminate costly downtime, and enhance quality control.
-
-
Featured
AltaML Secures Spot on AIFinTech100 for Consecutive Year
-
What We Do
-
Contact
-
Featured
Jurisage and CiteRight Finalize Merger, Become Jurisage Group Inc.
-
Services
-
What We Do
-
Feasibility AssessmentMinimize risk, maximize results, and set your organization up for success.
-
AI LabLeverage shared experience and collaboration to drive adoption and results.
-
Catalog of OpportunitiesEmbark on your AI adoption journey with confidence.
-
Generative AI WorkshopUncover innovative ways to engage with your data to shape your future.
-
-
-
Industries
-
Some Industries We Support
-
Energy and ResourcesDrive innovation and promote sustainability while gaining a competitive edge.
-
Financial ServicesBoost efficiency, reduce costs, and streamline processes.
-
Forestry and AgriculturePave the way for a productive, efficient, and greener future.
-
HealthRevolutionize care delivery, improve outcomes, and save lives.
-
ManufacturingStreamline production, eliminate costly downtime, and enhance quality control.
-
-
-
Venture Studio
-
What We Do
-
Contact
-
- Insights
-
About

Insights
The Data Shift: Readiness and Adoption in a Rapidly Changing Landscape

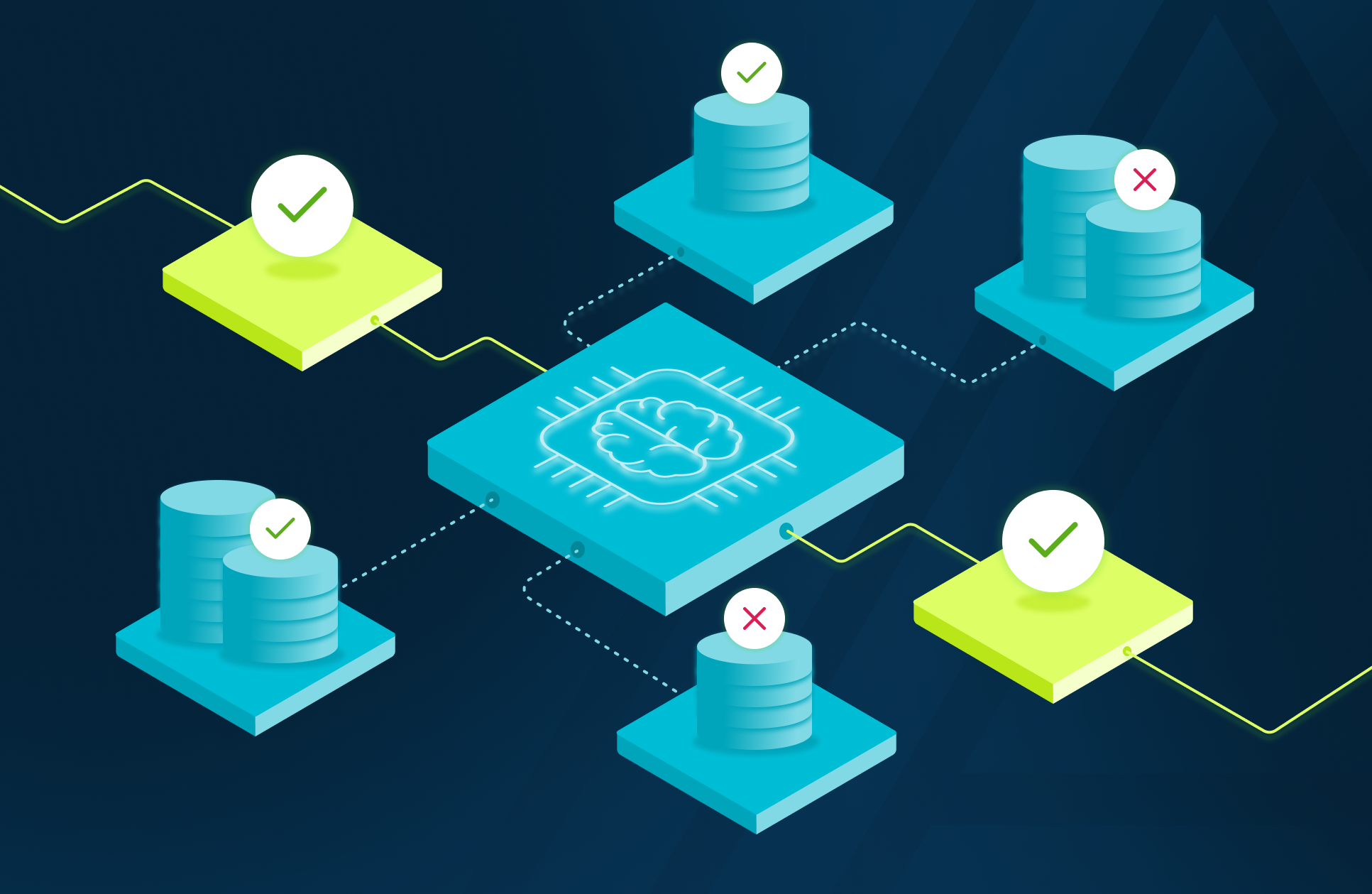
Artificial intelligence (AI) is a revolutionary force that will forever change the way companies do business. Globally, business leaders are aware of AI’s tremendous capacity for economic and social impact—but they are equally aware that history is full of boom and bust cycles, especially when it comes to purportedly disruptive technologies.
A certain degree of wariness toward early adoption of AI is understandable, if for no other reason than to avoid the misuse of resources i.e., time, money, and people into the complexities of building and deploying a usable AI model. Despite these concerns, companies realize the need to integrate AI into their businesses and are looking for ways to get started.
By the end of the decade, there will be two kinds of companies: 1. Those who fully utilize AI; and 2. Those who are out of business.
Peter Diamandis
Entrepreneur and Philanthropist
Still, when it comes to AI adoption for your organization, getting started now is often more important than having “perfect” data. In fact, if a company delays AI adoption for too long, there’s a serious risk it will lose its competitive edge to industry competitors who have begun experimenting with it already. In the realm of AI integration, the willingness to experiment holds greater value than the pursuit of perfectionism.
The Importance of Data for AI
Achieving data readiness for AI is the first stage in your AI journey. Collect data cohesively and with a clearly defined purpose, particularly considering the stringent constraints imposed by General Data Protection Regulation (GDPR) laws. Store your data in a universally usable format, ensuring it can be consistently accessed and collected across all data touchpoints and validated seamlessly when integrated into any product. Additionally, ensure that data remains readily available and accessible to all areas of your organization, whether it’s collected in a centralized storage system or flows through various isolated data points.
Establishing AI/ML Business Objectives First
To properly implement AI and machine learning (ML), it’s important to understand your enterprise needs by defining clear and measurable business objectives that could create value and in turn, profit.
Design your AI/ML model to tackle challenges that cannot be efficiently resolved using traditional rule-based methods or, at the very least, not with maximum efficiency. Duplication of purpose could hamper the efficiency of your teams, as adapting to a new mindset for handling a specific task will necessitate both time and effort. The first goal should be to start small, address the simple issues your company is facing to land some small wins. This will help get your team on board and open their eyes to the potential AI has to transform the rest of your organization. Further, your team can focus on definite key performance indicators (KPIs) that will provide immediate business value to your AI/ML model.
Defining a clear strategy based on immediate needs means attaining quick adoption and rapid deployment. Establishing overarching objectives that span across too many sectors will require alignment on multiple business units—an effort that may keep you from ever being ready to deploy. Having a clear and well-defined set of goals and needs helps you hone in on the data required, enabling you to begin leveraging your AI/ML solution quicker.
Data Governance and Scope for AI
One of the most relevant use cases for AI is the development of data governance tools and practices to jump over any potential pitfalls associated with modern data democratization trends. The need for trustworthy data means a lot of companies want to invest in data integrity. However, data usage costs are becoming unstable—to the point that 82% of data management decision-makers cannot forecast and control them anymore. This situation presents a significant challenge, wherein any effort towards data governance is accompanied by an inherent and unpredictable risk of potential cost escalation at any time.
AI plays a pivotal role in ensuring data governance without violating any industry-laid guidelines, such as GDPR or the Health Insurance Portability and Accountability Act (HIPAA). Not only does AI ensure data quality, but it’s becoming a near-mandatory entity in enforcing compliance. AI assists data governance and integrates itself with other assets such as business glossaries, policy engines, and orchestration engines. It establishes a fully-functional data governance ecosystem.
To effectively handle security, compliance, and legal concerns, it’s essential for the AI model to be in a fully operational state.In turn, this implies that data will only be fully compliant with governmental regulations after reaching this milestone. It’s a continuous cycle: data doesn’t achieve readiness until AI can effectively assist in its collection, and conversely, AI cannot be deployed until achieving data readiness. The strategy to overcome this challenge involves intensifying your endeavors to ensure data compliance during ML model development, and expediting AI deployment to enhance future data readiness, ultimately leading to its resolution.
Stay Ahead of the Curve
By dedicating time to AI/ML investments, you ensure the integration of a well-defined strategy that combines sufficient data readiness with achievable business goals. The extent of time your team devotes to data refinement and ML preparation directly correlates with the delay in your organization’s deployment readiness. This translates to dual financial setbacks for your business: increased development expenses due to prolonged workload, and a deferred start to harnessing the benefits of your solution deployment.