-
What We Do
-
Feasibility AssessmentMinimize risk, maximize results, and set your organization up for success.
-
AI LabLeverage shared experience and collaboration to drive adoption and results.
-
Catalog of OpportunitiesEmbark on your AI adoption journey with confidence.
-
Generative AI WorkshopUncover innovative ways to engage with your data to shape your future.
-
-
Featured
Why Enterprise AI Needs a Data-Product Approach
-
Some Industries We Support
-
Energy and ResourcesDrive innovation and promote sustainability while gaining a competitive edge.
-
Financial ServicesBoost efficiency, reduce costs, and streamline processes.
-
Forestry and AgriculturePave the way for a productive, efficient, and greener future.
-
HealthRevolutionize care delivery, improve outcomes, and save lives.
-
ManufacturingStreamline production, eliminate costly downtime, and enhance quality control.
-
-
Featured
AltaML Makes the List of Top FinTech Companies Leveraging Artificial Intelligence
-
What We Do
-
Contact
-
Featured
Jurisage and CiteRight Finalize Merger, Become Jurisage Group Inc.
-
Services
-
What We Do
-
Feasibility AssessmentMinimize risk, maximize results, and set your organization up for success.
-
AI LabLeverage shared experience and collaboration to drive adoption and results.
-
Catalog of OpportunitiesEmbark on your AI adoption journey with confidence.
-
Generative AI WorkshopUncover innovative ways to engage with your data to shape your future.
-
-
-
Industries
-
Some Industries We Support
-
Energy and ResourcesDrive innovation and promote sustainability while gaining a competitive edge.
-
Financial ServicesBoost efficiency, reduce costs, and streamline processes.
-
Forestry and AgriculturePave the way for a productive, efficient, and greener future.
-
HealthRevolutionize care delivery, improve outcomes, and save lives.
-
ManufacturingStreamline production, eliminate costly downtime, and enhance quality control.
-
-
-
Venture Studio
-
What We Do
-
Contact
-
- Insights
-
About
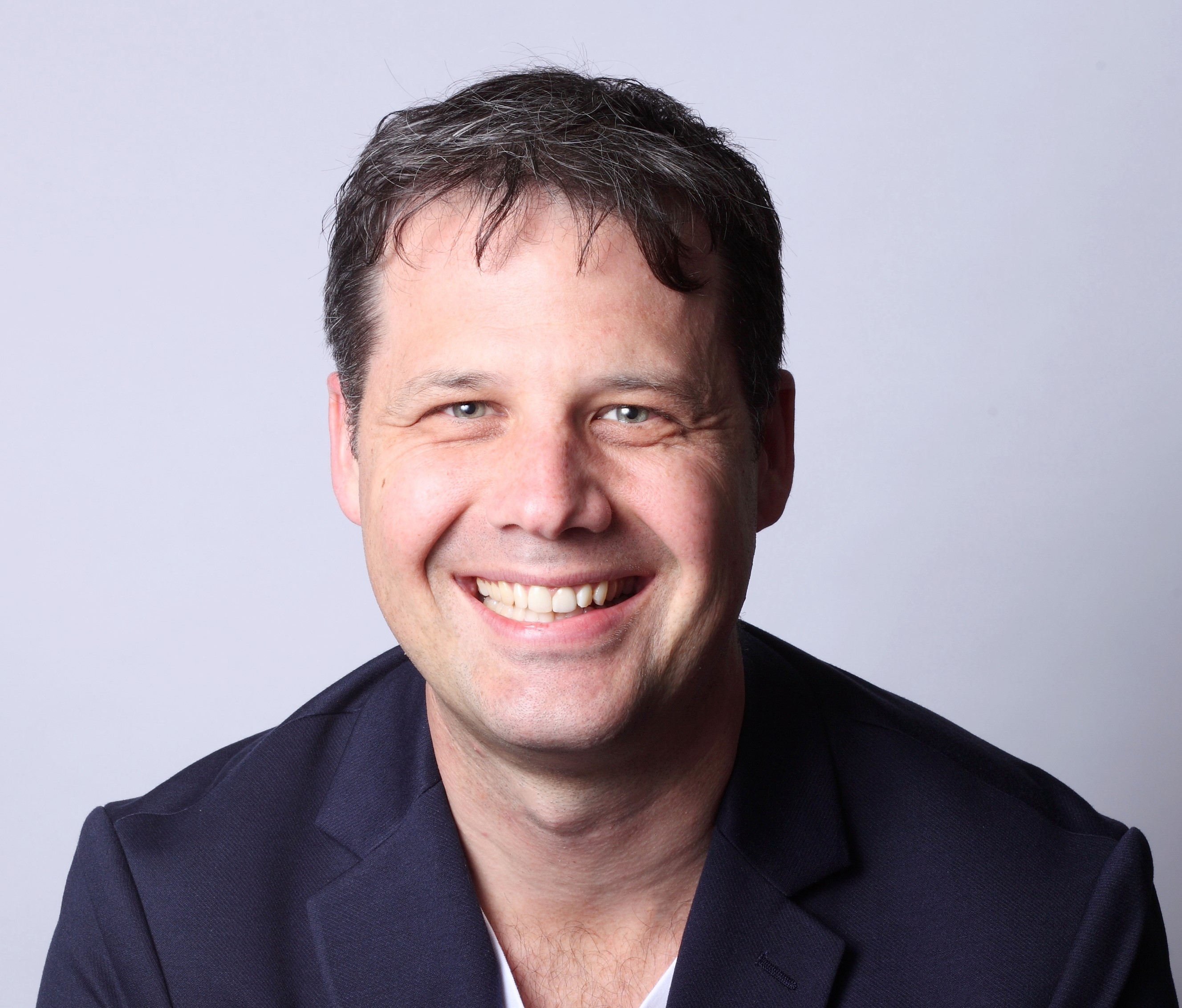
Insights
Decoding the Modern Approach to ML Adoption: Buy Vs. Build Vs. Partner
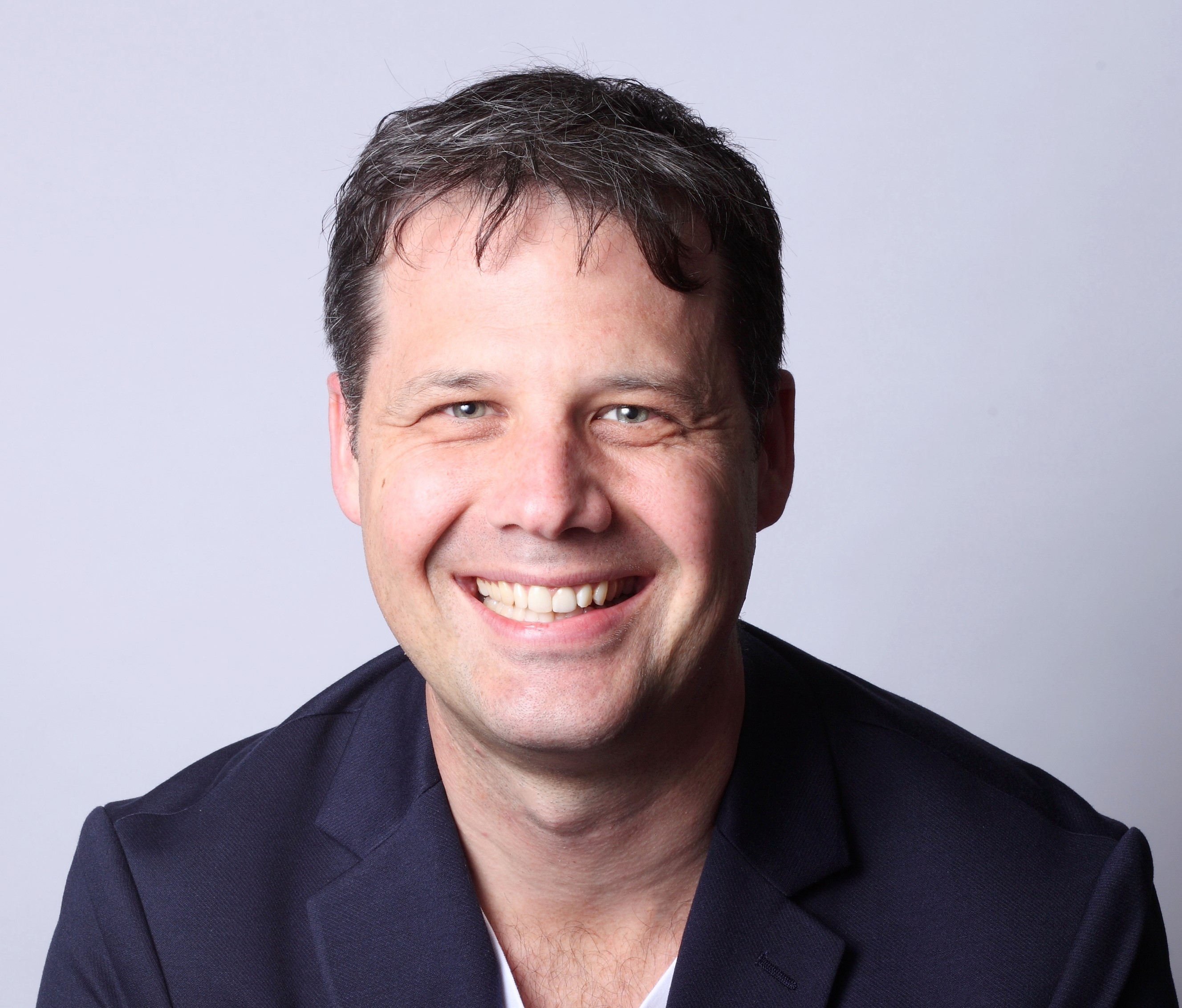
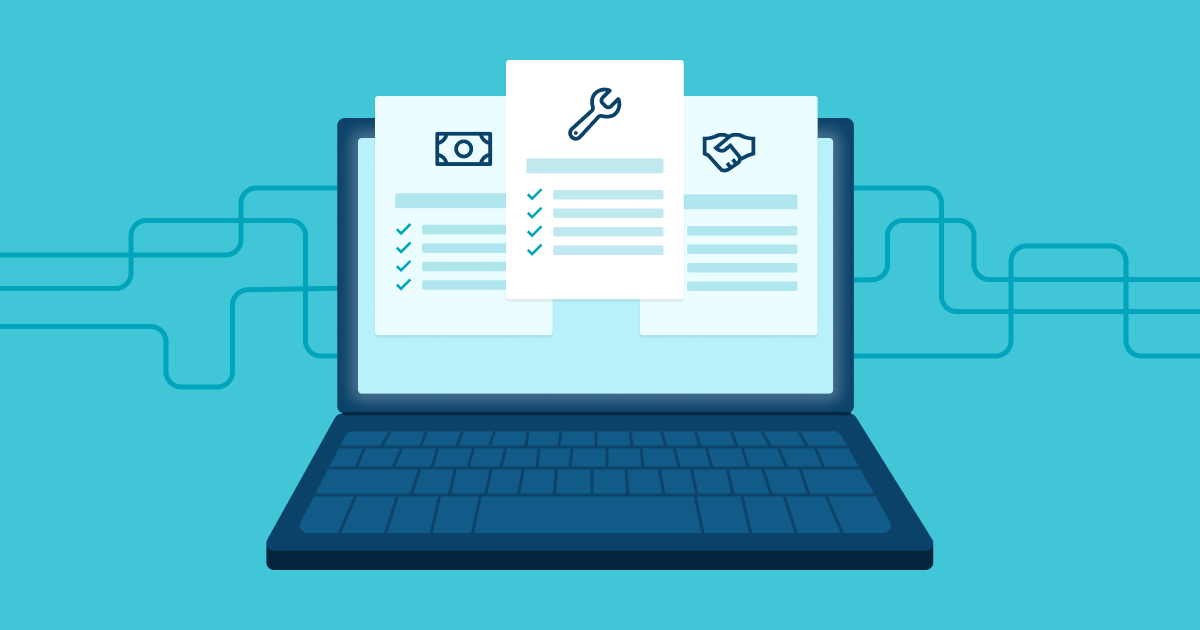
Very few technologies have proved to be as disrupting and revolutionary as artificial intelligence (AI) and machine learning (ML) solutions. Every major tech that defines our everyday living and highly interconnected world depends on some kind of ML infrastructure—from Netflix, and Google recommendations, to Facebook’s massive ad campaigns, to household gadgets such as Siri and Alexa.
On top of that, it’s no secret that ML-powered data analysis can provide a major competitive advantage in all industries. Nearly every company today has or plans to jump into ML to gain an edge over competitors. But how hard is that in practice?
Well, in a nutshell, very.
Any company essentially has three options:
- Buy: Purchasing an AI/ML solution that is compatible with your existing environment.
- Build: Establishing an in-house team and developing your own AI/ML solution.
- Partner: Collaborating with a third-party AI provider to introduce AI/ML within your organization.
Here’s a quick summary of the benefits and challenges of each solution:
Building Pros
- Increased Control: Building an AI team internally provides significant control over the development process. You can customize the team to your specific needs, set your own timelines, and make sure the team members are aligned with your company’s culture and goals. This control can lead to faster development and implementation of AI solutions.
- Ownership of Intellectual Property: In-house AI teams provide the advantage of owning the intellectual property (IP) they create, giving you a competitive edge by protecting your AI technology and preventing competitors from using it.
- Familiarity with Business Operations: An in-house AI team understands your company’s business operations, data, and customer needs better. They can develop solutions that are tailored to your specific industry and business, which can lead to better outcomes and greater return on investment (ROI).
Building Cons
- Cost: Building an AI team in-house can be expensive, as it requires hiring experienced data scientists, AI engineers, and ML experts. These professionals are in high demand and can command high salaries.
- Talent Shortage: The shortage of AI talent is a major challenge for companies looking to build an in-house AI team. There is intense competition for skilled AI professionals, and it can be challenging to find and retain top talent.
- Time-Consuming: Building an in-house AI team can be a time-consuming process. It can take many quarters to find and hire the right people, set up infrastructure, and develop the necessary skills and processes.
- Limited Perspective: An in-house AI team may have a limited perspective, as they are focused solely on your company’s operations. They may not have exposure to different industries, technologies, or approaches to AI development.
Buying Pros
- Time and Cost-Efficient: Purchasing an AI solution off-the-shelf can save a significant amount of time and money that would otherwise be spent on developing a custom solution.
- Established Performance: Off-the-shelf AI solutions have been tested and validated in the market. They have an established track record of performance, which can give you confidence in their effectiveness.
- Expert Support: AI vendors usually offer customer support and maintenance services that can provide technical assistance and support to ensure the solution is working optimally.
- Easy Implementation: AI solutions off-the-shelf are typically designed to be easy to implement and integrate with existing systems.
Buying Cons
- Limited Customization: Off-the-shelf solutions may not be tailored to your specific business needs, so they may lack certain functionalities that you require. Furthermore, there is a question of whether an off-the-shelf solution even exists for what you need to do. We’re still in the early stages of AI as an industry, and there often is not a canned solution.
- Compatibility Issues: AI solutions off-the-shelf may not be compatible with your existing systems, which can lead to issues with integration and performance.
- Security Risks: Purchasing an AI solution off-the-shelf may expose your business to security risks if the solution is not adequately secured against potential cyber threats.
- Lack of Control: With off-the-shelf AI solutions, you have limited control over the underlying technology, which may be a concern if you need to modify or adjust the solution in the future.
Partnering Pros
- Expertise: One of the primary advantages of using an outside provider for AI development is their expertise. Providers have experience working with different industries, technologies, and approaches to AI development. They can bring a fresh perspective and new ideas to the table, which can lead to better outcomes.
- Time Savings: Using an outside provider can save you time, as they have the necessary infrastructure, processes, and talent in place. They can start working on your project immediately and have the resources to complete it quickly.
- Flexibility: Outsourcing AI development provides flexibility. You can choose the provider that best fits your needs and can adjust the level of engagement as necessary. This can be particularly beneficial if you have a limited budget or a short-term project.
- Reduced Risk: When you use an outside provider, you transfer some of the risk associated with AI development to them. Providers are typically responsible for ensuring the quality of their work and meeting agreed-upon timelines.
- Access to Talent: Access to a multifaceted team who will help integrate AI into your business and plus you’re provided with an opportunity to upskill internal teams through educational sessions.
Partnering Cons
- Lack of Control: When you partner with a third-party AI provider, you are relying on their expertise and technology to develop and deploy AI solutions for your business. This can lead to a lack of control over the development process and the final product, which may not fully meet your needs or align with your business goals.
- Security Risks: Sharing sensitive data and information with a third-party AI provider can increase the risk of security breaches and data leaks. It is important to carefully vet any potential partners and ensure they have robust security measures in place to protect your data.
- Dependence on External Resources: When you partner with a third-party AI provider, you are relying on their resources and expertise to develop and deploy AI solutions for your business. This can make it difficult to make changes or updates to the solution without their involvement, leading to a lack of flexibility and potentially higher costs.
- Cost: Partnering with a third-party AI provider can be expensive, especially for ongoing maintenance and support. It is important to carefully consider the costs involved and ensure that the benefits of partnering outweigh the financial investment.
- Integration Challenges: Integrating third-party AI solutions with existing systems and processes can be complex and time-consuming. This can lead to delays and additional costs, as well as potential disruptions to business operations. It is important to carefully consider the integration requirements and ensure that any potential partners have experience with integrating their solutions with similar systems.
The Case for Buying
Nearly 80% of enterprises admitted to preferring to buy a pre-built AI application or just develop a proof-of-concept, according to a 2021 IBM survey. This is just another piece in a puzzle demonstrating that very few companies have the resources to build their own AI solutions, let alone deploy them and realize ROI. Despite investing in ML solutions, seven out of 10 companies have reported no economic value, rendering ROI a mirage.
So why does this happen?
Building requires developing an ML infrastructure, training it, building an entire team to put it in place and then finding its niche so it can become profitable. Hiring data scientists is costly, given that the salary for a mid-level one is $130,000 on average. Not to mention acquiring this talent can be difficult. By 2025, it’s projected that growing job demand will result in 97 million jobs working directly with AI. However, a recent Deloitte report showed 68% of executives are already facing moderate to extreme skills shortages within their organizations.
Moreover, building an AI solution is a very intensive operation, requiring significant organizational efforts to develop the new processes required to manage the ML project. An organization’s data scientists and ML engineers may require their own project manager to coordinate their team efforts, and the ML solution must be tried and tested by various executives with no prior experience in this field before it can be deployed.
Finally, building is always a time-intensive adventure. We live in a frantic and constantly changing world; by the time a built-in-house ML solution is ready to be rolled out, there’s a good chance it could be obsolete.
The Case for Building
Buying a pre-trained ML model and software package seems like an easy solution for companies who don’t want to devote a large portion of their budget to building and deploying a new one. However, things are hardly so simple in reality.
Buying certainly seems like the easiest route, it’s the one that focuses on the lightest and least expensive lift and yet it often proves to be the most rigid, least efficient alternative. Although some degree of flexibility can be provided in the form of updates and improvements for the tool, any off-the-shelf ML platform is nothing but a one-size-fits-all solution that can hardly provide the exact answer to every organization’s question. In other words, although the returns would certainly be immediate, organizations won’t realize a business impact as effective as a “build” or “partner” alternative. An anemic result may very well defeat the whole point of implementing a complex ML solution.
And that’s not to mention all those instances where buying a solution off-the-shelf is simply not possible due to the highly specific needs of a use case. Customization is, in some instances, a requirement rather than a luxury. One example of this is the medical imaging industry: the sheer amount of different data formats used in this niche is too broad for a readily available ML tool to handle them all.
Security is another barrier to buying ML solutions. Buying a commercial ML platform means having to accept the security standards of that provider—which may be insufficient if the data handled is sensitive or highly valuable.
The Case for Partnering
So, building an ML solution is often too time-intensive and provides little-to-no ROI. Buying an ML solution provides quicker returns, but off-the-shelf AI is seldom secure or specific enough to the needs of a use case.
Partnering is a very pragmatic approach that involves working side-by-side with a third-party AI provider to create a customized ML solution. Essentially, partnering involves buying something that you build and because of this approach, you don’t have to deal with the cons of either.
Instead of having to hire an entire team of data analysts, engineers, and developers from scratch, a partner already has everything you need to build a solid ML platform—from expertise to hardware. Therefore, partners are fully dedicated in providing the best AI services as part of their mission; they will be able to help your company understand how ML deployment may act as a strategic differentiator while avoiding the risks of developing a solution that does not fit well with your existing resources or business goals.
By leveraging the capabilities of a fully developed AI solution, collaboration between the company and third-party partners will allow your business to compete in its own vertical.
Our Recommendation: Hybrid Approach
To develop an effective AI solution, a hybrid approach combining internal team-building with partnering with a third-party AI provider is recommended. This way, companies can leverage the benefits of both approaches and scale their AI initiatives more efficiently while building out their internal capabilities over time.
Building an internal AI team provides companies with complete control over the development process and enables them to create a customized solution that aligns with their specific needs and goals. However, building a team from scratch can be time-consuming and resource intensive. Additionally, it may be difficult to find the necessary talent, especially in highly competitive industries.
Partnering with a third-party AI provider can help to address these challenges. By choosing a partner who is experienced and dedicated to providing the best AI services, companies can leverage their expertise and resources to create a solid ML platform without having to hire an entire team of data analysts, engineers, and developers from scratch. This approach allows companies to focus on their core competencies while still benefiting from the expertise of the partner.
Conclusion
In conclusion, there are various factors to consider when deciding between buying an AI solution off-the-shelf, building an internal AI team, partnering with a third-party AI provider or choosing a hybrid approach. Ultimately, the right choice depends on your business goals, resources, and risk tolerance. A thoughtful evaluation of these factors can help you make an informed decision that best serves your business needs.
So you might ask, what approach best suits your company? That’s ultimately up to you.