-
What We Do
-
Feasibility AssessmentMinimize risk, maximize results, and set your organization up for success.
-
AI LabLeverage shared experience and collaboration to drive adoption and results.
-
Catalog of OpportunitiesEmbark on your AI adoption journey with confidence.
-
Generative AI WorkshopUncover innovative ways to engage with your data to shape your future.
-
-
Featured
Navigating Bias in AI with Open-Source Toolkits
-
Some Industries We Support
-
Energy and ResourcesDrive innovation and promote sustainability while gaining a competitive edge.
-
Financial ServicesBoost efficiency, reduce costs, and streamline processes.
-
Forestry and AgriculturePave the way for a productive, efficient, and greener future.
-
HealthRevolutionize care delivery, improve outcomes, and save lives.
-
ManufacturingStreamline production, eliminate costly downtime, and enhance quality control.
-
-
Featured
AltaML Secures Spot on AIFinTech100 for Consecutive Year
-
What We Do
-
Contact
-
Featured
Jurisage and CiteRight Finalize Merger, Become Jurisage Group Inc.
-
Services
-
What We Do
-
Feasibility AssessmentMinimize risk, maximize results, and set your organization up for success.
-
AI LabLeverage shared experience and collaboration to drive adoption and results.
-
Catalog of OpportunitiesEmbark on your AI adoption journey with confidence.
-
Generative AI WorkshopUncover innovative ways to engage with your data to shape your future.
-
-
-
Industries
-
Some Industries We Support
-
Energy and ResourcesDrive innovation and promote sustainability while gaining a competitive edge.
-
Financial ServicesBoost efficiency, reduce costs, and streamline processes.
-
Forestry and AgriculturePave the way for a productive, efficient, and greener future.
-
HealthRevolutionize care delivery, improve outcomes, and save lives.
-
ManufacturingStreamline production, eliminate costly downtime, and enhance quality control.
-
-
-
Venture Studio
-
What We Do
-
Contact
-
- Insights
-
About

Insights
Navigating Bias in AI with Open-Source Toolkits

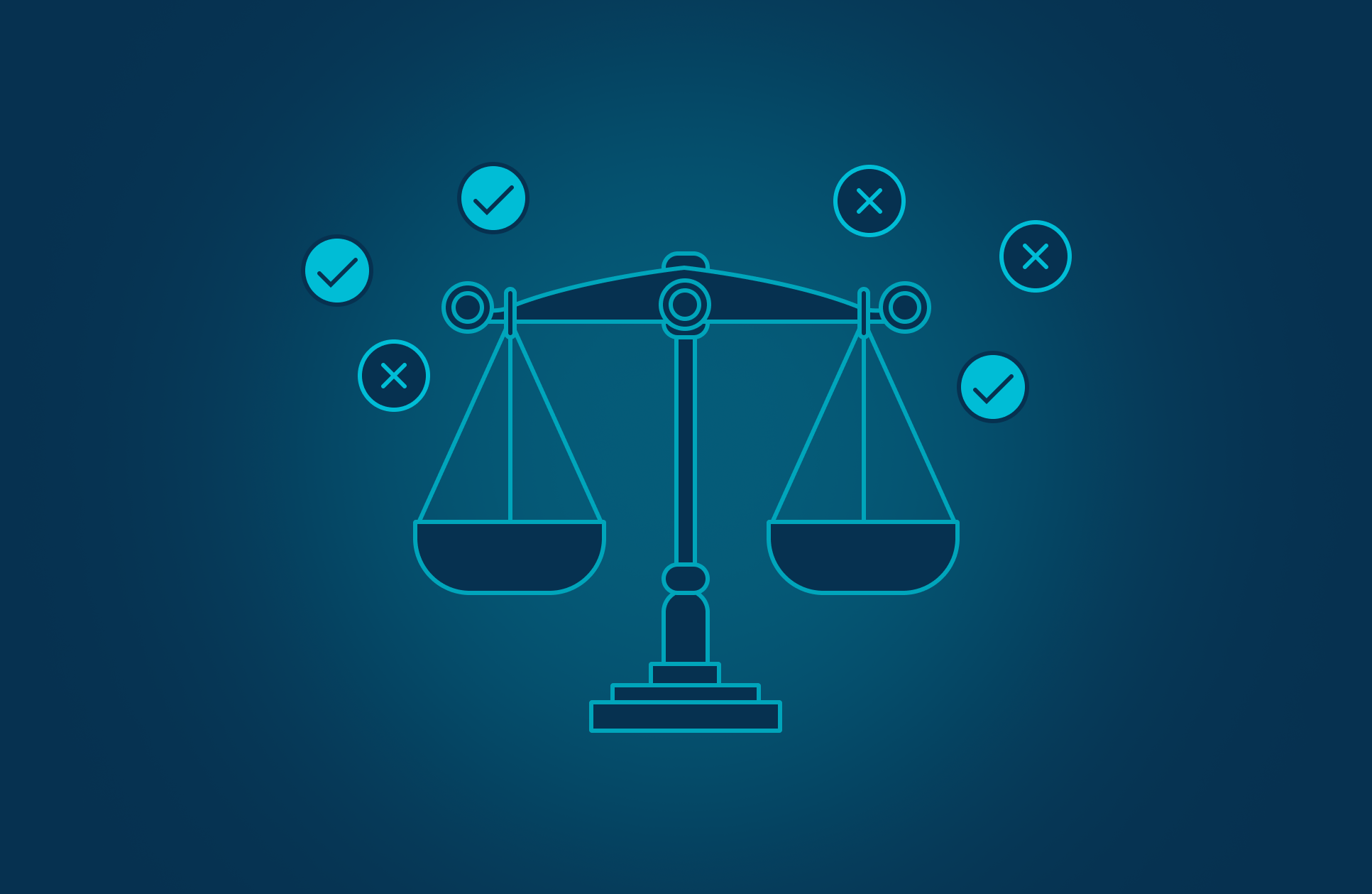
In an era of rapid technological advancement, the integration of artificial intelligence (AI) and machine learning (ML) has catalyzed revolutionary changes across industries, offering opportunities for data-driven decision-making. However, these advancements have also brought forth ethical concerns, notably the pressing issue of harmful bias in AI systems.
Harmful bias refers to biases within AI systems that result in unfair or discriminatory treatment toward individuals or groups. Incidents involving harmful biased AI algorithms, such as Amazon’s attempted deployment of a compromised HR tool and Northpointe’s discriminatory COMPAS system, have underscored the ethical dilemmas posed by unchecked biases in AI. In response to these challenges, open-source toolkits have emerged providing promising options for researchers and practitioners to identify, explain, and mitigate bias in AI systems.
Here we look into the potential of leveraging existing open-source toolkits to address bias in AI and offer recommendations to promote ethical AI practices.
AI Bias Amplifies Human Bias
Bias and discrimination are deeply ingrained in the historical fabric of human societies. The incorporation of AI into decision-making processes heightens the risk of biased outcomes, as illustrated in the figure below:
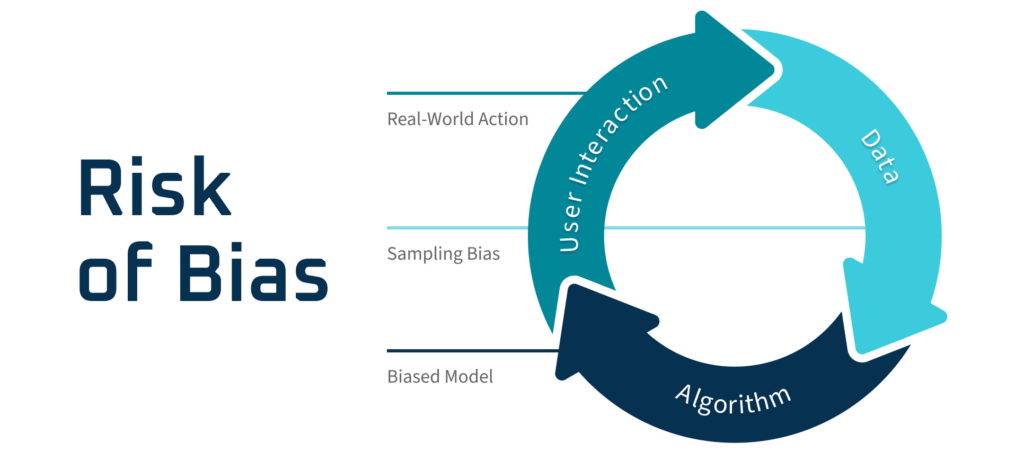
AI biases manifest in various forms, such as allocation harms and quality of service harms (Table 1), which can perpetuate existing inequalities and undermine fairness. Examples like the COMPAS and Amazon’s HR tool cases illustrate how AI biases can impact real-world outcomes, underscoring the urgency of addressing bias in AI systems.
Allocation Harms | This situation arises when AI systems either extend or withhold opportunities, resources, or information selectively for certain individuals or groups. |
Quality of Service Harms | This refers to instances where AI systems exhibit disparities in performance across different demographic groups, leading to unequal levels of service quality. An example is when a facial detection model effectively recognizes faces of certain races but struggles with others. |
In recent times, there has been a growing emphasis on tackling bias issues, with a particular focus on identifying two primary types: data biases and model biases. Data biases, originating from skewed or incomplete datasets, encapsulate inherent prejudices within the data. These biases can be further amplified by model biases, which stem from the algorithms and decision-making processes utilized.
Tackling Bias with AI Toolkits
To effectively combat harmful biases, open-source toolkits have emerged, providing practitioners with a range of metrics and techniques to assess fairness in AI systems. Notable examples include IBM’s AI Fairness 360, Microsoft’s Fairlearn, Google’s What-If Tool, and Aequitas. These toolkits have greatly facilitated efforts to address bias in AI by offering comprehensive frameworks for identifying and mitigating harmful biases.
Steps to Mitigate Bias:
- Identify: Biases in machine learning can be grouped into two types: data biases and model biases. The first step is to identify data biases, which can be challenging due to limited access to protected variables such as gender, race, and age. Statistical tests like the chi-square test or group representation metrics available in open-source toolkits like AIF360 can be used for this purpose.
- Explain: Once biases are identified, the next step is to analyze and understand how features present in the data contribute to the bias. Explainable AI tools, including but not limited to visualization techniques like partial dependence plots and Individual Conditional Expectation (ICE) plots, can help interpret model outcomes and explain biases.
- Mitigate: Techniques for mitigating bias fall into four categories: pre-processing, in-processing, post-processing, and meta techniques. Pre-processing involves adjusting the dataset before training, such as reweighting samples to address biases. In-processing techniques are integrated into the learning process itself, such as debiasing with adversarial learning. Post-processing involves modifying the outputs of the model after training, such as threshold optimization to ensure fairness. Additionally, meta techniques, such as grid search over hyperparameters with respect to bias metrics, provide a higher-level approach to bias mitigation. These techniques are commonly implemented in open-source toolkits to help promote fairness in AI systems.
- Communicate: Effective communication throughout the model-building process is crucial. Tools like Google Model Card toolkits can assist with documentation and communication during the handoff of AI models.
Available Toolkits
Toolkits | FairLearn | What-If Tool | AIF-360 | Aequitas |
Highlight | Best in Functionality | Can identify the most similar cases to compare predictions | The first comprehensive tool with more than 70 metrics | Most user-friendly with a decision tree provided to assist metrics selection |
Language Options | Python | Python | Python and R | Python |
Assessment and Mitigation | Both | Both | Both | Assessment only |
Open Source Visualization Dashboard | Yes | Yes | No | Yes |
Who Maintains It? | Microsoft | IBM | School researchers | |
Notes | Define fairness in relation to potential harm | Requires data upload, no analysis on-premise which can raise privacy concerns | Focus on debiasing | Tool not as well maintained |
Conclusion
The integration of open-source toolkits presents a promising approach to combat bias in AI systems. By leveraging these toolkits and adopting best practices for ethical AI, stakeholders can mitigate biases, enhance transparency, and promote fairness in AI-driven decision-making.
However, challenges remain. These challenges include the limited coverage of multi-classification and regression use cases, inconsistencies in terminology, and a lack of awareness among AI practitioners, particularly regarding access to protected variables crucial for effective bias mitigation. For example, collecting demographic data specifically for this purpose is not a common practice, presenting a significant hurdle in addressing bias effectively.
It is recommended that AI practitioners, users, and corporations prioritize the documentation of data sources, adherence to ethical principles, and transparent communication regarding bias mitigation strategies. At AltaML, we recognize the importance of ethical ML practices and are deeply committed to addressing bias in AI systems. Learn more about AltaML’s practices in responsible AI here.